The rise of AI-enhanced credit scoring systems marks a significant shift in how financial institutions assess creditworthiness. Traditional credit scoring models have long been constrained by a limited set of factors, often excluding individuals without formal credit histories. However, AI-based models leverage a broader range of data sources, including non-traditional metrics such as social media activity, online purchases, and utility payments. This approach provides a more accurate and efficient means of evaluating credit risk, particularly in emerging markets.
In economies undergoing rapid digital transformation, data has become a powerful tool for reshaping credit-scoring foundations. Traditional methods, reliant on stringent norms, have inadvertently excluded large segments of the underbanked population. Many of these individuals lack access to formal banking systems or credit histories. With internet connectivity and smartphone usage proliferating, AI-driven solutions are beginning to close this gap. Incorporating non-traditional data metrics such as utility payments and network usage, AI-based credit scoring models offer a more inclusive and comprehensive assessment of creditworthiness, especially for those previously underserved by traditional financial systems.
This transformative shift not only broadens financial access but also enables institutions to build more precise and adaptable risk models, making AI-enhanced credit scoring a critical innovation in the future of financial services.
Understanding AI-based Credit Scoring
AI-based credit scoring is an advanced method of evaluating an individual’s creditworthiness using artificial intelligence (AI) and machine learning (ML). Unlike traditional credit evaluation, which primarily considers factors like credit history, income, and debt, AI-based models analyze a wider range of data sources, including digital footprints. Utilizing complex algorithms, the models assess and predict future financial behavior, offering deeper insights that help lenders make more informed decisions. This approach also enables the evaluation of individuals without conventional credit histories, by analyzing alternative data such as online transactions, social media activity, and mobile app usage.
Mechanism Behind AI-Based Credit Scoring
AI-based credit scoring is an innovative approach that allows for a more dynamic, comprehensive, and accurate evaluation of a borrower’s creditworthiness. Let’s understand how AI-based credit scoring works:
#1 Data Collection and Analysis
The initial phase of AI-based credit scoring involves comprehensive data collection. In traditional models, the focus is mainly on credit history. Whereas AI systems can process and evaluate a wide range of data sources. These include bank transactions, bill payments, social media activity, and mobile phone usage patterns. Such extensive data collection provides a holistic view of an individual’s financial behavior, offering deeper insights into their creditworthiness.
#2 Machine Learning Models
At the heart of AI-based credit scoring are machine learning models. These models are trained on large datasets to identify patterns and correlations that may indicate a borrower’s likelihood of repaying a loan. By examining thousands of data points, machine learning algorithms reveal subtle relationships between seemingly unrelated factors and a person’s financial reliability, enhancing the overall assessment process.
#3 Predictive Analytics
Once trained, the machine learning model is employed for predictive analytics. When a new credit application is submitted, the AI system evaluates the applicant’s data against the patterns identified during training. It then generates a score that forecasts the applicant’s creditworthiness based on a complex analysis of multiple factors, including those that may not be immediately apparent, even to financial experts.
#4 Continuous Learning
A significant advantage of AI-based credit scoring is its ability to learn and adapt over time. As the system processes more applications and gathers feedback on its predictions—such as whether borrowers fulfill their loan obligations—it can refine its models for enhanced accuracy. This continuous learning ensures that the AI system remains attuned to evolving financial behaviors and market conditions, ultimately leading to better credit assessments.
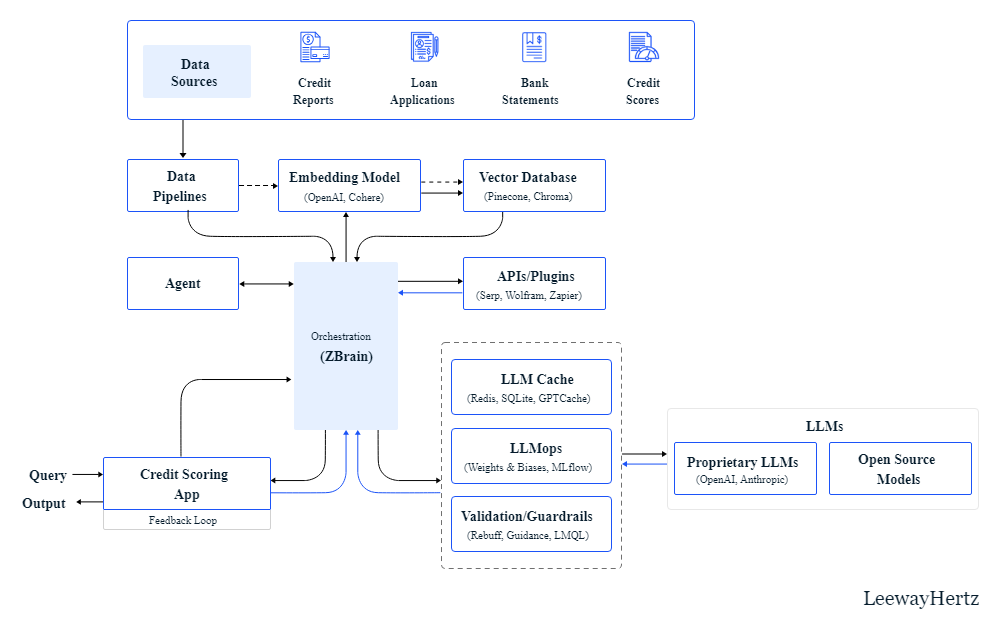
Key Applications of AI-Driven Credit Scoring
AI-powered credit scoring enhances the efficiency, accuracy, and decision-making processes for banks and fintech firms. Lenders are increasingly adopting AI to evaluate the creditworthiness of both individuals and businesses. Below are some of the most impactful applications of AI-based credit scoring in finance and lending.
Accelerating Loan Approvals
AI plays a crucial role in loan approval processes, whether for personal or business purposes. By analyzing vast datasets, including transaction histories, social media activity, and user behavior, AI can extract meaningful insights for loan approval decisions. This accelerates loan processing times and ensures that qualified individuals receive approvals swiftly and seamlessly.
Comprehensive Data Analysis
Traditional credit scoring systems often fail to assess individuals with little or no credit history, relying solely on historical financial data. In contrast, AI algorithms can evaluate both traditional data, such as credit history, and alternative sources, including cash flow, utility payments, and rental history. This holistic approach allows lenders to gain a more accurate understanding of a borrower’s current and future financial stability.
Streamlining Credit Card Issuance
AI-based credit scoring models are increasingly being used in credit card issuance. These models assess applicants’ credit history, debts, income, and financial behavior, enabling financial institutions to offer personalized credit limits and promotional offers. This leads to more informed decisions regarding credit risk assessment.
Calculating Insurance Premiums
These models offer a comprehensive view of financial responsibility, extending beyond credit history, income, and debts. By predicting repayment reliability, these algorithms help insurance providers determine premiums based on an individual’s financial health and risk profile, enhancing the accuracy of premium assessments.
Promoting Financial Inclusion
AI-driven credit scoring models are instrumental in advancing financial inclusivity. Unlike traditional systems that exclude individuals with no formal credit history, AI evaluates a broader range of data to extend credit to underserved populations, including the unbanked, young adults, and immigrants. This opens up access to financial services, promoting broader economic participation.
How AI-Powered Credit Scoring Enhances Risk Assessment
AI in credit scoring offers a significant improvement over traditional scorecard-based risk assessment models that rely heavily on historical borrowing behavior.
Real-Time and Dynamic Assessment: AI-based credit scoring takes a more adaptive approach, evaluating borrowers in real time. Instead of relying solely on historical data, AI models consider a range of dynamic factors, such as current income, employment status, and future earning potential. This allows financial institutions to assess borrowers based on their current financial position and future prospects, enabling greater inclusion in credit programs.
Risk Management Through Behavioral Analysis: AI systems can identify risky borrowing behaviors that may not be flagged by traditional models. For instance, frequent credit card churning or sudden changes in spending patterns may indicate higher risk, allowing lenders to make more informed decisions. Conversely, borrowers who may not have an extensive credit history but demonstrate strong earning potential are more likely to be approved under AI-powered assessments.
Improved Predictive Capabilities: AI-driven credit scoring models provide more accurate predictions by analyzing real-time data from diverse sources. This broader evaluation gives financial institutions a clearer picture of a borrower’s financial behavior, allowing for better risk management and more precise lending decisions.
Addressing Challenges in AI Credit Scoring: Transparency and Bias Mitigation
While AI-driven credit scoring offers advanced predictive capabilities, it faces key challenges related to model transparency and decision bias. Ensuring clarity in machine learning processes and fairness in outcomes is crucial for widespread adoption.
Tackling the “Black Box” Problem
One major concern with AI models is their opacity, often referred to as the “black box” problem. This lack of transparency makes it difficult to understand how decisions are made. To address this, mechanisms have been developed that enhance explainability at both the individual and model-wide levels. These tools enable financial analysts to identify which features drive credit scoring decisions, ensuring a clearer understanding of how the model functions.
Visualizing Credit Default Risk Predictions
Advanced visualization tools have been introduced to demystify AI-driven predictions. By illustrating the cumulative impact of various features on credit default risk, analysts can see how different factors influence model outputs. Color-coded visualizations, for instance, help clarify the relationship between feature values and their contribution to risk assessments, offering an intuitive way to interpret complex AI models.
Model Score Distribution Visualization
To further enhance understanding, Model Score Distribution plots provide a detailed visualization of output scores across target classes. This allows for a comprehensive view of score distribution, giving analysts a clearer picture of how predictions align with real-world creditworthiness.
Mitigating Bias in AI Credit Scoring
To ensure fairness, AI credit scoring models must address potential bias. A stratified modeling approach, along with manual weighting of data points, helps minimize bias and ensures that no demographic or regional group is disproportionately affected. This approach maintains the objectivity of the scoring system, reducing the risk of biased outcomes.
The Bottom Line
The future of AI-based credit scoring is poised for ongoing advancements and greater adoption throughout the financial sector. As machine learning algorithms grow more sophisticated and alternative data sources become increasingly accessible, these AI-driven credit scoring models are anticipated to achieve enhanced accuracy and comprehensiveness in evaluating credit risk.
The application of AI in credit scoring allows for the evaluation of creditworthiness for individuals who may lack a traditional credit history. Instead, it assesses various alternative data footprints, including online transactions, social media interactions, browsing behavior, and mobile app usage.
Read More: AP Automation Adoption: The Productivity Paradox
[To share your insights with us, please write to psen@itechseries.com ]